Meta unveils AI tools to give robots a human touch in physical world
Meta unveils three tools and benchmarks to enhance robot touch perception, dexterity, and human-robot collaboration in real-world settings. …
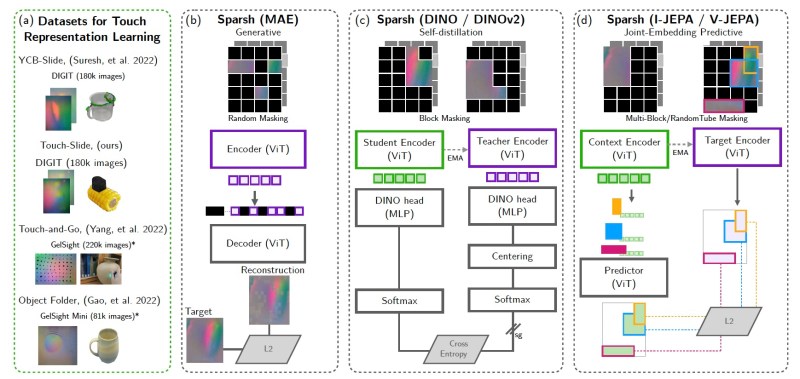
Join our daily and weekly newsletters for the latest updates and exclusive content on industry-leading AI coverage. Learn More
Meta made several major announcements for robotics and embodied AI systems this week. This includes releasing benchmarks and artifacts for better understanding and interacting with the physical world. Sparsh, Digit 360 and Digit Plexus, the three research artifacts released by Meta, focus on touch perception, robot dexterity and human-robot interaction. Meta is also releasing PARTNR a new benchmark for evaluating planning and reasoning in human-robot collaboration.
The release comes as advances in foundational models have renewed interest in robotics, and AI companies are gradually expanding their race from the digital realm to the physical world.
There is renewed hope in the industry that with the help of foundation models such as large language models (LLMs) and vision-language models (VLMs), robots can accomplish more complex tasks that require reasoning and planning.
Tactile perception
Sparsh, which was created in collaboration with the University of Washington and Carnegie Mellon University, is a family of encoder models for vision-based tactile sensing. It is meant to provide robots with touch perception capabilities. Touch perception is crucial for robotics tasks, such as determining how much pressure can be applied to a certain object to avoid damaging it.
The classic approach to incorporating vision-based tactile sensors in robot tasks is to use labeled data to train custom models that can predict useful states. This approach does not generalize across different sensors and tasks.

Meta describes Sparsh as a general-purpose model that can be applied to different types of vision-based tactile sensors and various tasks. To overcome the challenges faced by previous generations of touch perception models, the researchers trained Sparsh models through self-supervised learning (SSL), which obviates the need for labeled data. The model has been trained on more than 460,000 tactile images, consolidated from different datasets. According to the researchers’ experiments, Sparsh gains an average 95.1% improvement over task- and sensor-specific end-to-end models under a limited labeled data budget. The researchers have created different versions of Sparsh based on various architectures, including Meta’s I-JEPA and DINO models.
Touch sensors
In addition to leveraging existing data, Meta is also releasing hardware to collect rich tactile information from the physical. Digit 360 is an artificial finger-shaped tactile sensor with more than 18 sensing features. The sensor has over 8 million taxels for capturing omnidirectional and granular deformations on the fingertip surface. Digit 360 captures various sensing modalities to provide a richer understanding of the environment and object interactions.
Digit 360 also has on-device AI models to reduce reliance on cloud-based servers. This enables it to process information locally and respond to touch with minimal latency, similar to the reflex arc in humans and animals.

“Beyond advancing robot dexterity, this breakthrough sensor has significant potential applications from medicine and prosthetics to virtual reality and telepresence,” Meta researchers write.
Meta is publicly releasing the code and designs for Digit 360 to stimulate community-driven research and innovation in touch perception. But as in the release of open-source models, it has much to gain from the potential adoption of its hardware and models. The researchers believe that the information captured by Digit 360 can help in the development of more realistic virtual environments, which can be big for Meta’s metaverse projects in the future.
Meta is also releasing Digit Plexus, a hardware-software platform that aims to facilitate the development of robotic applications. Digit Plexus can integrate various fingertip and skin tactile sensors onto a single robot hand, encode the tactile data collected from the sensors, and transmit them to a host computer through a single cable. Meta is releasing the code and design of Digit Plexus to enable researchers to build on the platform and advance robot dexterity research.
Meta will be manufacturing Digit 360 in partnership with tactile sensor manufacturer GelSight Inc. They will also partner with South Korean robotics company Wonik Robotics to develop a fully integrated robotic hand with tactile sensors on the Digit Plexus platform.
Evaluating human-robot collaboration
Meta is also releasing Planning And Reasoning Tasks in humaN-Robot collaboration (PARTNR), a benchmark for evaluating the effectiveness of AI models when collaborating with humans on household tasks.
PARTNR is built on top of Habitat, Meta’s simulated environment. It includes 100,000 natural language tasks in 60 houses and involves more than 5,800 unique objects. The benchmark is designed to evaluate the performance of LLMs and VLMs in following instructions from humans.
Meta’s new benchmark joins a growing number of projects that are exploring the use of LLMs and VLMs in robotics and embodied AI settings. In the past year, these models have shown great promise to serve as planning and reasoning modules for robots in complex tasks. Startups such as Figure and Covariant have developed prototypes that use foundation models for planning. At the same time, AI labs are working on creating better foundation models for robotics. An example is Google DeepMind’s RT-X project, which brings together datasets from various robots to train a vision-language-action (VLA) model that generalizes to various robotics morphologies and tasks.